Sustained growth and success in the dynamic environment of non-contractual enterprises depend heavily on the understanding and optimization of Customer Lifetime Value (CLV). CLV is the total amount of money a customer brings into your company over the course of their relationship. By concentrating on this indicator, businesses can better allocate resources, customize their marketing plans, and increase client retention. It might be difficult to calculate CLV accurately in sectors like digital agency, eCommerce, and retail. Predictive analytics, on the other hand, provides a potent way to find untapped revenue streams and improve corporate performance.
To forecast future client behavior, predictive analytics makes use of data, statistical algorithms, and machine learning approaches. Data analysts and BI teams don’t need to be highly skilled in machine learning to uncover significant insights thanks to no-code predictive analytics SaaS platforms.
It is especially useful to forecast future purchases, next buy dates, and future purchase quantities in non-contractual firms, where client relationships are frequently more flexible. Businesses can effectively engage and keep customers by using focused marketing tactics that anticipate customer behavior by leveraging predictive analytics capabilities.
For non-contractual firms trying to maximize their marketing and sales efforts, customer lifetime value is crucial. Businesses can determine new income prospects and evaluate CLV with the aid of predictive analytics. Businesses can develop customized tactics that promote client retention, raise CLV, and eventually spur growth by utilizing data.
What is Customer Lifetime Value?
Customer Lifetime Value (CLV) is a critical metric that helps businesses gauge the long-term worth of each customer. It represents the total revenue a customer is anticipated to bring to your business throughout their entire relationship. Calculating CLV involves adding historical purchases to future predicted purchases, providing a comprehensive view of the customer’s value.
By understanding CLV, businesses can make well-informed decisions and develop strategies that boost their bottom line.
Customer Lifetime Value (CLV) is a metric that quantifies the total revenue a customer is expected to generate for a business throughout their relationship. In non-contractual businesses, such as retail, eCommerce, and digital agencies, customers are not bound by long-term contracts or subscription agreements. As a result, CLV assessment becomes a critical element for these businesses to understand customer behavior, optimize marketing efforts, and enhance customer retention.
In non-contractual settings, customers can switch between providers, making their purchasing patterns less predictable. Consequently, accurately estimating CLV becomes even more critical as it helps businesses allocate resources more effectively and identify high-value customers. By focusing on CLV, non-contractual businesses can target their marketing campaigns, design personalized offers, and drive customer loyalty.
Evaluating CLV in non-contractual businesses poses several challenges, including:
- Irregular purchase patterns: Unlike contractual businesses, where customers make recurring payments, non-contractual customers often exhibit sporadic and inconsistent purchasing behavior. This inconsistency makes it difficult to predict future revenue and estimate CLV accurately.
- Limited data: Non-contractual businesses often have fewer customer data to work with compared to their contractual counterparts. This lack of data hampers the ability to make informed decisions and effectively target high-value customers.
- Higher customer acquisition costs: Due to the absence of long-term contracts, non-contractual businesses usually face higher customer acquisition costs. As a result, accurately assessing CLV becomes vital to ensure that marketing efforts generate a positive return on investment (ROI).
- Varying customer behavior: In non-contractual industries, customers may have diverse preferences, behaviors, and expectations. This variation makes it challenging to develop a one-size-fits-all approach to estimate CLV and design targeted marketing campaigns.
Predictive analytics plays a pivotal role in overcoming the challenges associated with assessing CLV in non-contractual businesses. Predictive analytics can help businesses forecast future customer behavior, estimate revenue potential, and enhance marketing efforts by harnessing the power of data, machine learning, and statistical algorithms. Here’s how predictive analytics addresses the challenges of CLV assessment in non-contractual businesses:
- Predicting purchase patterns: With predictive analytics, businesses can analyze historical customer data to identify patterns and trends in purchasing behavior. This insight enables them to predict future purchases, estimate the next purchase dates, and forecast future purchase amounts more accurately.
- Utilizing available data: Predictive analytics can help non-contractual businesses maximize the limited data available. By leveraging advanced algorithms and machine learning models, companies can extract valuable insights from existing customer data and make informed decisions.
- Optimizing marketing ROI: Predictive analytics allows businesses to identify high-value customers and allocate marketing resources effectively. Businesses can optimize marketing ROI and reduce customer acquisition costs by targeting customers with a higher propensity to buy or those who are likely to churn.
- Personalizing marketing efforts: Predictive analytics helps businesses segment their customers based on behavior, preferences, and demographics. This segmentation allows them to design personalized marketing campaigns that resonate with individual customers, ultimately driving customer loyalty and increasing CLV.
Understanding and assessing Customer Lifetime Value is vital for non-contractual businesses looking to optimize their marketing strategies and enhance customer retention. Despite the unique challenges these businesses face, predictive analytics offers a powerful solution that enables them to predict customer behavior, allocate resources efficiently, and maximize revenue. By leveraging tools of no-code predictive analytics, data analysts and BI teams can uncover valuable insights and make data-driven decisions that improve CLV and fuel business growth.
Non-contractual businesses face unique challenges when it comes to assessing Customer Lifetime Value. However, predictive analytics offers a powerful solution that enables these businesses to predict future customer behavior, allocate resources effectively, and maximize revenue.
One of the most valuable insights predictive analytics can provide is an estimate of the number of future purchases a customer is likely to make. By analyzing historical data and customer behavior patterns, businesses can predict which customers are more likely to make repeat purchases and those at risk of churning. No-code predictive analytics platform simplifies this process by offering pre-built models that help data analysts and BI teams without ML expertise make accurate predictions.
With a future number of purchases prediction, businesses can:
- Identify high-value customers and develop personalized marketing campaigns to retain and upsell them.
- Optimize resource allocation by focusing on customers with the highest potential CLV.
- Detect early signs of customer churn and take proactive measures to re-engage them.
How do You Calculate Customer Lifetime Value?
The customer lifetime value calculation is not a straightforward step-by-step process. There’s more than one way to discover the value of your average customer.
In this section, we will discuss the different ways you can put an accurate dollar figure on your customers.
Calculating CLV: general formula
To begin, the most basic customer lifetime value formula of all is as follows:
Customer lifetime value = (customer value x average customer lifespan)
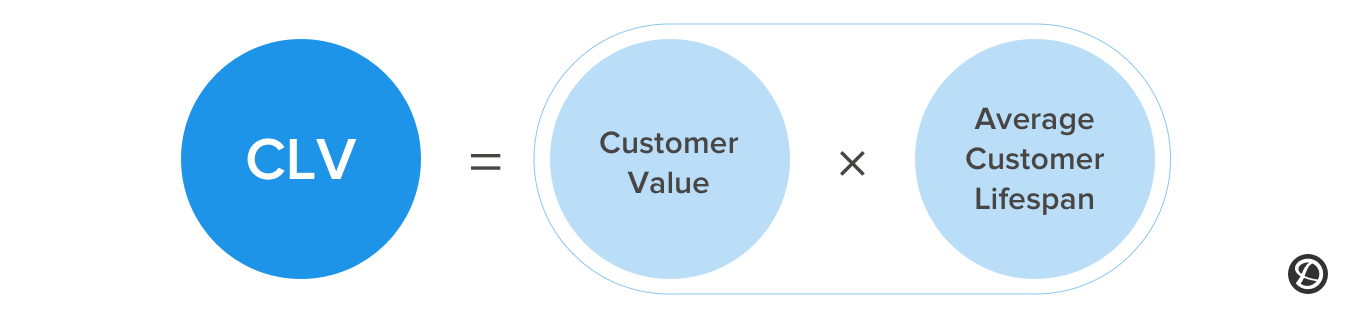
The simplest way to find the right numbers to plug into this formula is to calculate the average purchase value of your customers and multiply that number by the average number of purchases.
For the second part of the formula, you need to dive into your figures and calculate how long the average customer has a relationship with your brand.
Once you have these two figures, multiply them together to get the lifetime value of your customers.
Let’s look a bit deeper at each of the components of the customer lifetime value formula next.
Lifetime value formulas
You might choose to use different calculations for your CLV for various reasons. It’s useful for businesses who want to re-examine their customer retention strategies or gauge the financial viability of their operations. True data-driven companies don’t predict what customers find valuable about their brands. They find the truth.
Read Also: Real-time Data Analytics in Marketing Automation
To calculate Customer Lifetime Value, you’ll need to calculate total customer value first.
Total customer value
To find the total value of your customers, you’ll need to calculate the average purchase value (or how much a customer spends on an average order) and the average frequency rate first. Calculate those two variables with the formulas below before multiplying the average purchase value by your average purchase frequency rate.
Total customer value = (average purchase value x average frequency rate)
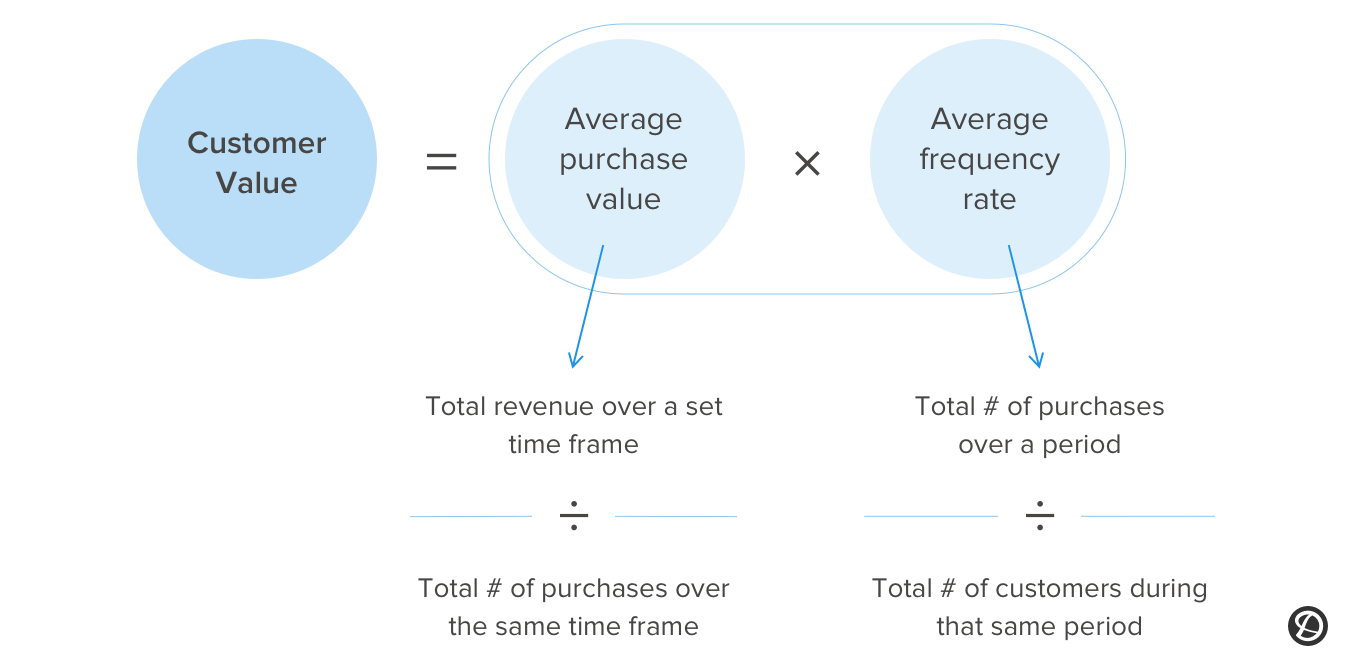
You might find that the value of your customers is higher or lower than you think. Comparing value to previous periods can be extremely helpful for tracking how you’re serving your existing customer base.
Average purchase value
If you want to find out the average purchase value for your business, you need to divide the total revenue of your company over a set period by the number of purchases over the same period.
Average purchase value = (total revenue over a set time frame / number of purchases over the same time frame)
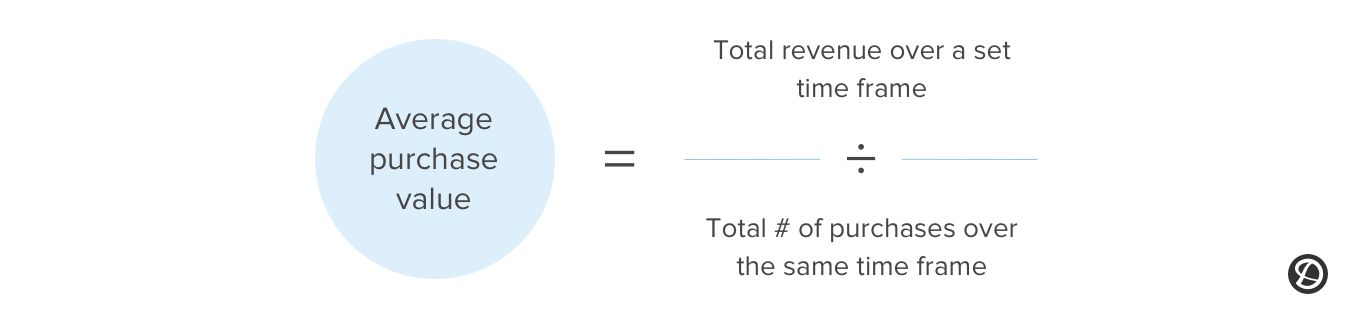
Most companies will use a year’s worth of data, but you can also do it by quarter. Finding this number allows you to tell how much the average customer spends on each purchase. It’s also an easy way to find out how big your customer’s baskets are.
Average purchase frequency rate
Finding the average purchase frequency rate tells you how often your customers are making a purchase, giving you some insight into consumer buying behavior and how you might be able to influence it. To find this number, divide the total number of purchases over a period by the unique number of customers.
Average purchase frequency rate = (total number of purchases over a period / number of customers during that same period)
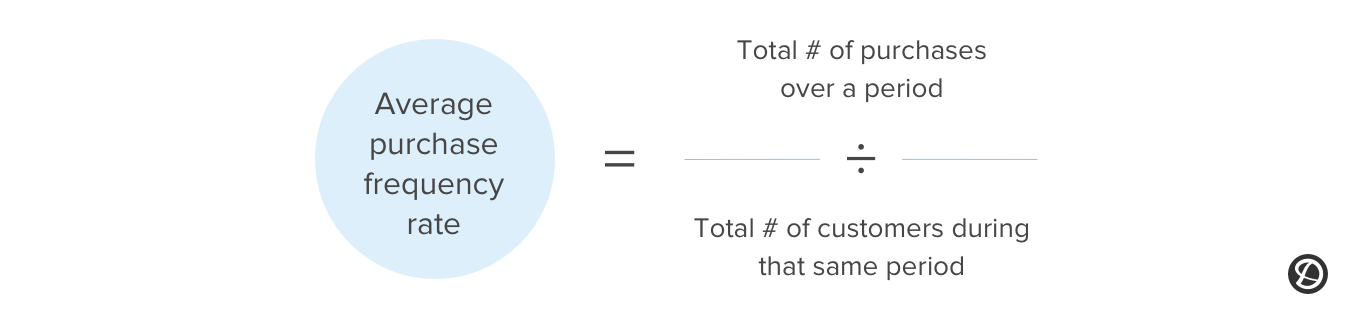
Average customer lifespan
Customer retention is essential to the success of any business. The longer you hold a customer, the greater their lifetime value. Approximately 82% of companies say that retention is more valuable than acquisition.
To work out the average customer lifespan, take the average number of years a customer stays active and divide it by the total number of customers.
Average customer lifespan = (average number of years a customer stays active / number of customers)
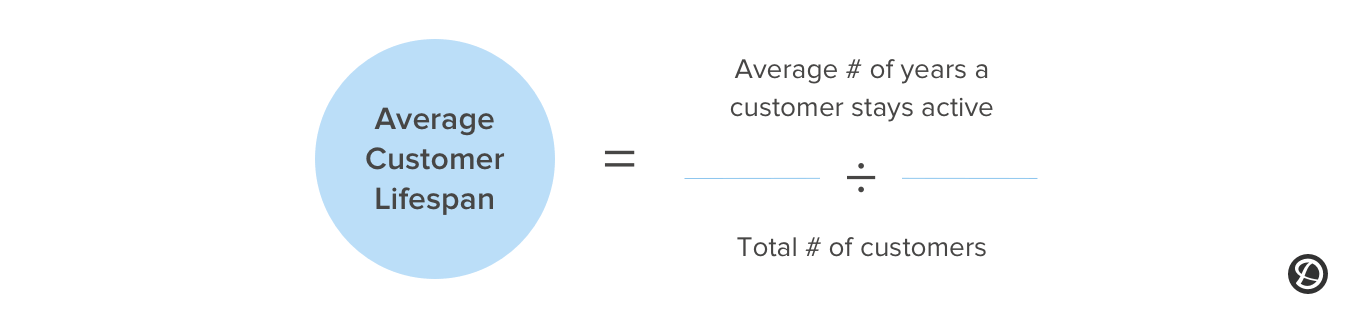
Once you have the above components calculated, you can enter the values into the comprehensive customer lifetime value formula outlined below.
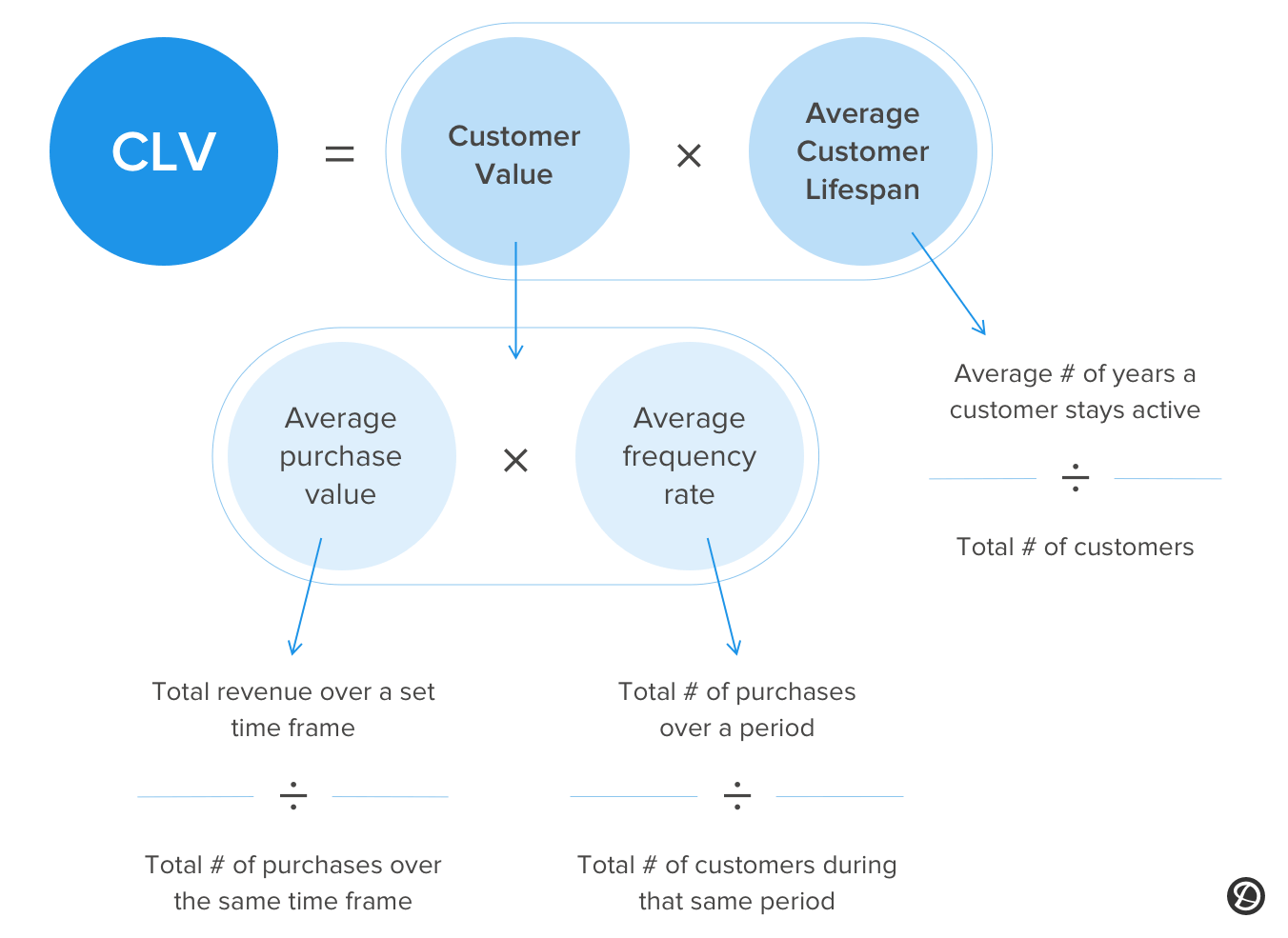
Customer lifetime value models
There are two models you can use to measure the value of your customers during the relationship. Deciding on one model over the other will lead to different outcomes.
Your chosen model depends on whether you want to predict the future using current and past customer behavior or use historical numbers to make an accurate prediction.
Predictive model
The predictive model forecasts future lifetime value by considering the buying behaviors of new and existing customers. These calculators use a combination of machine learning and regression.
The advantage of using the predictive model is identifying your most valuable customers. This figure can also show you which products and services are responsible for the most revenue, which can help you improve your customer retention strategy.
Historical model
The historical model is the simplest because it uses past data to predict customer value. It also doesn’t consider whether your existing customers will be customers in the future.
Businesses that only interact with their customers for a set period will find this model most useful. However, since the customer journey is different for everyone, the historical model does have its drawbacks. Active customers could become inactive and cause inaccurate figures. It also works the other way, with inactive customers becoming active again.
To measure and improve your CLV, you need to collect and analyze data from various sources, such as your website, CRM, email, social media, and surveys. Utilizing tools and platforms that integrate and visualize your data, like Google Analytics, HubSpot, or Tableau, can help you track key performance indicators (KPIs) like CLV, CAC, ROI, retention rate, and net promoter score.
To further optimize your CLV, you should consistently monitor and adjust your marketing activities based on the insights from the data. Additionally, segmenting customers based on their value and needs to create buyer personas can be beneficial. Crafting value propositions that differentiate you from competitors can also be an effective strategy.
Building relationships with customers through content marketing and providing them with value-added services is a great way to increase trust. Offering discounts, bundles, subscriptions, loyalty programs, and referral programs are all great ways to encourage more purchases. Finally, asking for feedback and reviews from customers can help you better understand their needs and complaints.
To use CLV to optimize your marketing campaigns, you need to first understand the customer journey and the factors that influence their purchase decisions. Then, you can use this data to create campaigns that attract and acquire new customers with a high potential CLV, nurture and educate existing customers with a low or medium CLV, retain and delight loyal customers with a high CLV, and reactivate and win back lost customers with a high CLV.
You can do this by providing relevant content, offers, and support that address customer pain points, rewarding loyal customers with exclusive benefits and discounts, and reaching out to lost customers with personalized messages, incentives, and feedback requests. By doing so, you can increase customer satisfaction and loyalty while restoring trust.